Blog
Webinar Recap: Generative AI for OSINT – 4 Next-Level Techniques
Check out our recap of the four open-source intelligence (OSINT) use cases outlined in our recent webinar with SANS Institute Instructor, Matt Edmondson.
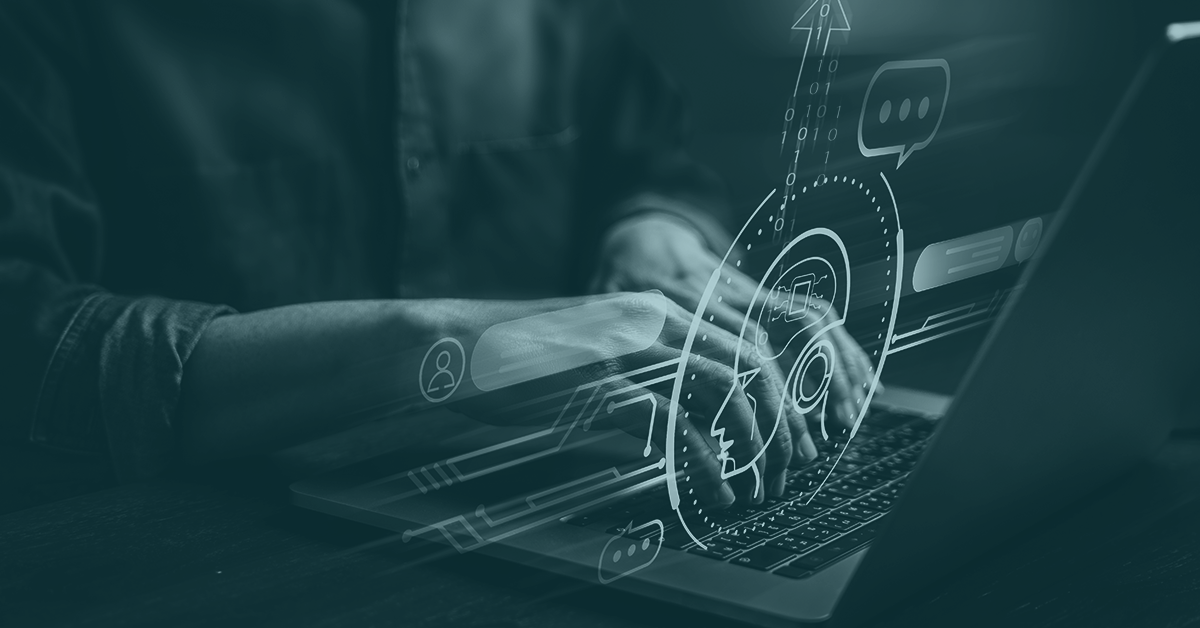
In recent years, the artificial intelligence landscape, particularly large language models (LLMs) such as ChatGPT, has undergone a remarkable transformation. With advancements in model size, data volume, and training techniques, these models are now capable of delivering results that were once unimaginable. Last week, Flashpoint, accompanied by Matt Edmondson, delved into some significant improvements that have propelled generative AI to the forefront of innovation.
Significant improvements to ChatGPT
One of the standout enhancements to ChatGPT is its built-in capability to retrieve real-time information and data from the web. It also now includes functionality for analyzing and summarizing documents provided by users, extracting valuable insights with unparalleled efficiency.
Asking ChatGPT itself about improvements made to its system, the chatbot generated the following graph:
AI techniques
1. Persona creation
Persona creation is a versatile tool in the OSINT arsenal, enabling analysts to navigate digital landscapes, interact discreetly, and extract valuable intelligence while safeguarding anonymity and operational security. ChatGPT’s ability to assume diverse personas was demonstrated with striking accuracy, offering useful insights into different online identities.
Matt asked ChatGPT to assume the persona of a young male cyber criminal in Russia with strong technical skills who uses these skills to make money and does not need to worry about legal consequences. He then struck up a casual conversation with it, and the results were uncannily accurate and believable:
2. Code analysis
In OSINT, code analysis is pivotal in identifying security threats, software vulnerabilities, and emerging technologies. Leveraging ChatGPT’s capabilities, analysts can streamline the code analysis process, saving valuable time and resources, particularly for less experienced developers.
Here, Matt produced a simple Python script that can analyze a Python program and concisely report its functionality:
The finished report contained an analysis of the program, including what it does, how it does, and operational security concerns.
This capability can save an incredible amount of time when reviewing code, especially for less experienced developers.
3. Train offline models with your own data
Offline large language models (LLMs) offer a compelling solution for organizations requiring training on proprietary data while maintaining operational security. By harnessing the power of LLMs, such as NVIDIA’s “Chat With RTX,” organizations can achieve remarkable accuracy and depth of insight.
In the webinar, we showcased this using NVIDIA’s “Chat With RTX” program to obtain information about Juan Soto. Initially, RTX knew very little about the baseball player or his team, and provided outdated, inaccurate information about his abilities.
However, after uploading a 300-page PDF of baseball statistics, we asked the same question a few minutes later. This time, the results were 100% accurate and incredibly detailed.
After learning about Soto, the program even provided advice on whether the player was a good draft pick.
4. Grounding: Improving accuracy through real-world input
Ground truth, supplemented by input from individuals with firsthand knowledge, is essential for ensuring the accuracy and relevance of intelligence gathered. Innovations in frameworks like CrewAI and Microsoft’s AutoGen enable the development of applications with multiple AI agents, enhancing quality control and accuracy.
Frameworks like CrewAI and Microsoft’s AutoGen enable users to develop applications with multiple AI Agents.
Now, organizations can use one agent to research information online, another agent to do the work, and another to run quality control and make recommendations on improving it before presenting it to the user.
Multiple AIs can be used to fact-check and run quality control on each other. Two instances help because they are both imperfect—working together to deliver more accurate results.
Unlock new capabilities with Flashpoint
Advancements in ChatGPT and other LLMs are revolutionizing the OSINT landscape, empowering analysts with unparalleled capabilities. By embracing these innovations, organizations can confidently navigate complex digital environments, extracting actionable intelligence to inform critical decision-making processes.
Watch the full webinar recording for a deeper look into the latest AI tools and techniques for OSINT.